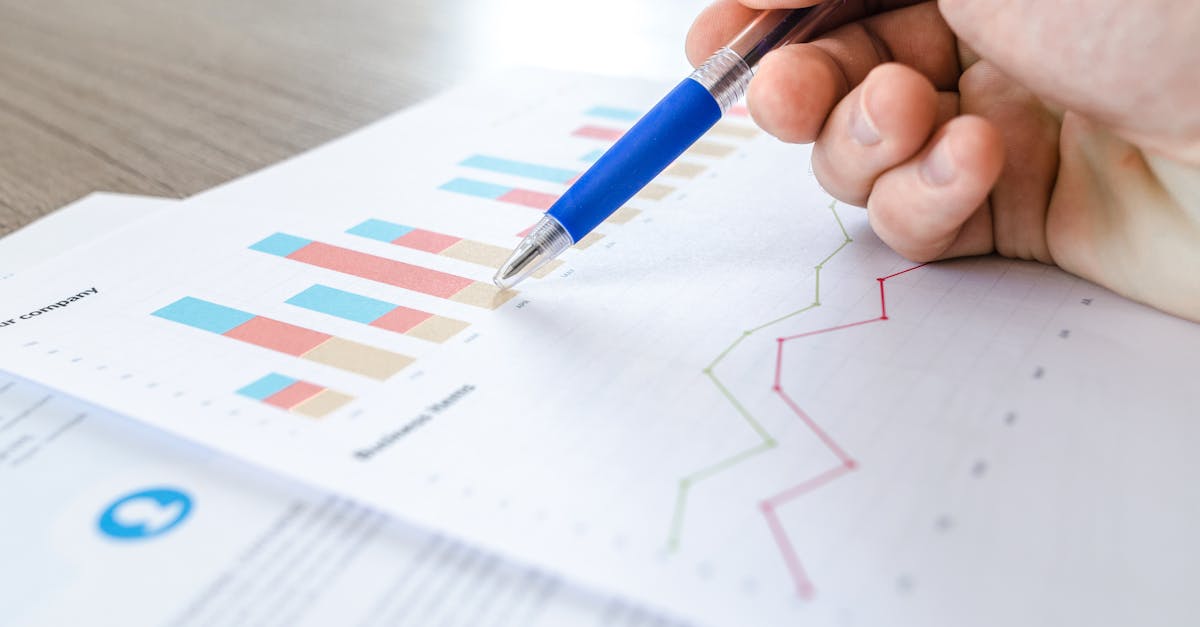
What does sensitivity mean in statistics?
One of the key concepts in statistics is the sensitivity of statistical tests and models. The sensitivity of a statistical test is how likely it is to reject the null hypothesis when the statistical model is correct. For example, a model is more sensitive if it is more likely to detect a true relationship between two variables when the relationship exists than when it doesn’t.
What does sensitivity mean in statistics workbooks?
You may have also heard the term “statistical sensitivity” used in the context of a statistical analysis. Sensitivity refers to the impact a small change in the data has on the statistical results. If the data is more variable than normal, sensitivity increases. The opposite is true if the data is less variable than normal. Statistically sensitive experiments are more likely to show a significant result when the data is closer to the hypothesized value than when the data is farther away from the hypothesized value.
What does sensitivity mean in statistics term?
Statistics is the branch of science that deals with the analysis and evaluation of data to prove the existence of relationships and make accurate predictions. Statistics is a form of mathematics where the number of variables and outcomes are generally very large. A sensitivity analysis is a statistical evaluation and comparison of the results when the input data or model assumptions are slightly changed. It shows how the results of a statistical analysis change with small variations in the initial data or input assumptions.
What does sensitivity mean in statistics test?
You can use the sensitivity of a statistical test to figure out if the results of your analysis are likely due to chance. A test’s sensitivity refers to the probability that the test will correctly detect a significant result if there is a true relationship between the variables you are testing.
What is sensitivity in statistics text?
We can also look at the sensitivity of a statistical test. If a test rejects the null hypothesis at a low probability level, the observed data might just be due to random chance. If we want to detect as little as possible chance as the cause of the observed results, we need to increase the power of the test. One way is to decrease the probability level at which the test rejects the null hypothesis. This is called the sensitivity of the test. A lower probability level increases the sensitivity of the test